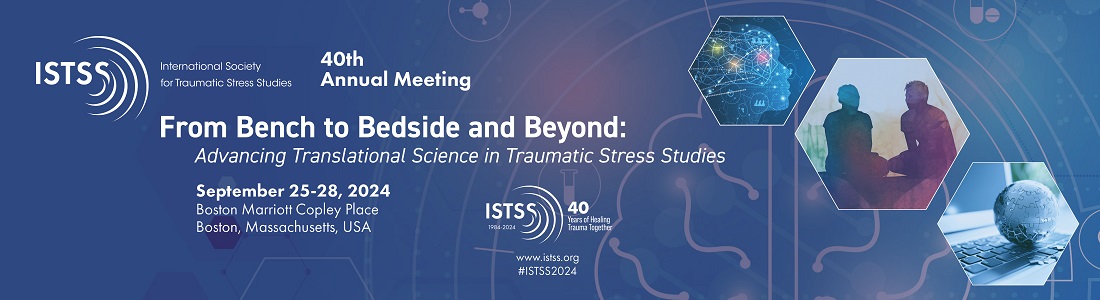
Master Methodologists
Innovations and Applications of Large Language Models (LLM)Â and Emerging AI Technologies
In a futuristic revival of the "Master Methodologist" Invited Speaker sessions longtime ISTSS Annual Meeting attendees may remember from past conferences, two researchers at the forefront of technology and health shed light on the ways that large language modeling and other cutting-edge developments in artificial intelligence are impacting the study and treatment of traumatic stress.Â
Large Language Models (LLM) and other Natural Language Processing (NLP) methods have emerged as tools to improve the study and measurement of stress. Through the analysis of unstructured text data (including therapy transcripts, medical records, and social media), researchers can study linguistic markers of stress, promising both predictive and monitoring capabilities of symptoms levels.
Part 1: Applications of NLP Methods and LLMs
The speakers will elucidate fundamental NLP approaches—including lexicon methods, topic modeling, word vectors, and deep learning models—and their significance to stress research by reviewing findings from the literature. Understand potential sources of clinical text data and explore areas of applications, including the study of patient and provider characteristics.
Part 2: State-of-the-Art LLM Architectures and Their Emergent Properties
Learn about the training steps to align an LLM to the medical domain—specifically, the capabilities of a medically aligned LLM (Med-PaLM 2) at assessing the diagnosis and symptoms of stress- and trauma-related conditions. The speakers will discuss the potential to augment LLM inputs with data from wearable devices and medical sensors that enable models to process numerical physiological and behavioral data in a more principled manner—a process known as "grounding." Lastly, explore considerations to take into account when using LLMs as a tool from a clinician or patient prospective.